Resource allocation for Multi-Tenant Retrieval Augmented Generation Systems
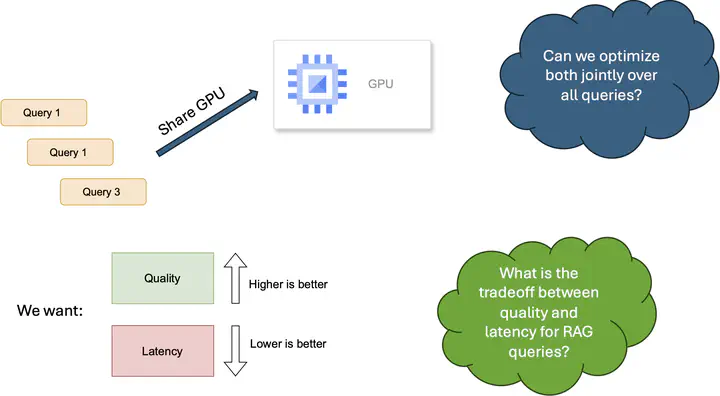
Retrieval Augmented Generation (RAG) is the recent state-of-the art paradigm which lets Large Language Models (LLMs) generalise to text generation tasks in new domains, for which they have not been trained. Traditionally, this generalisation was achieved by fine-tuning the LLM on a small amount of in-domain data, which is slow and expensive, as compared to RAG.
RAG today focuses on two key ideas information retrieval or how to fetch the relevant chunks to answer the LLM query and synthesis or how to combine the chunks for the LLM to process efficiently. In this paper, we show how the choice of configurations can have a significant trade-off between generation quality and system resource utilization in the synthesis section of the RAG pipeline. We conduct a preliminary measurement study to show how different configurations can yield the an optimal method for combining the retrieved documents to obtain the best performance in terms of output quality and output latency, while also measuring the effect of the trade-offs in choosing one dimension over the other.